Case Study:
Predictive Maintenance
Business Goals:
Anticipate equipment failure.
Increase predictive maintenance.
Reduce reactive service calls by 30%.
Outputs & Benefits:
Variables & patterns most related to future failure;
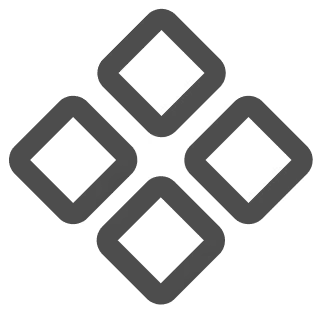
Provides engineering insight into failure points.
Probability of failure within various time periods.
Model statistics like precision, recall, false positive rates, etc.
Anticipation & reduction of service calls:
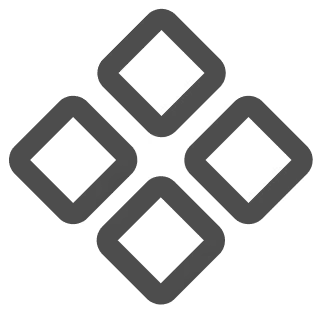
Calls reduced by 30+%.
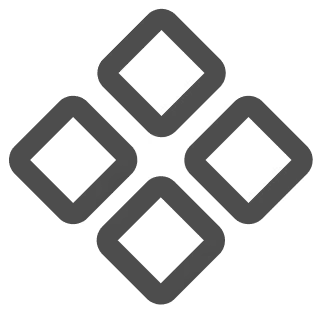
Costs reduced by 20+%.
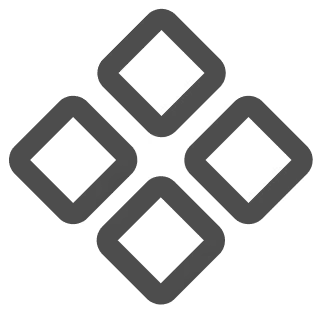
Many on-demand calls transformed into anticipatory ones.

Data Sets & Models:
Log data from equipment.
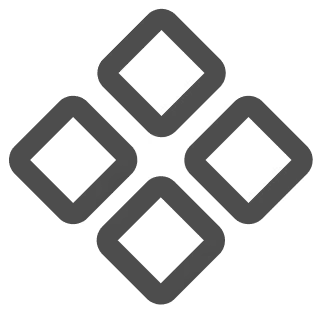
Sensor readings.
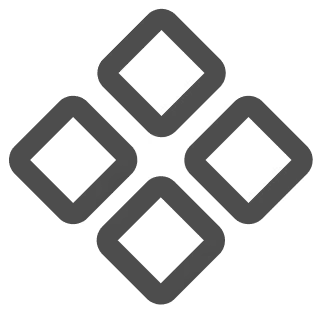
Error, warning, and status codes.
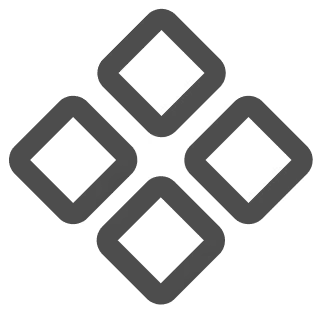
Failure flag.
Machine ID#'s and diagram of manufacturing process.
Both random forests & neural network models trained on data.